What the AI Impact on Data Engineering Jobs Looks Like Right Now
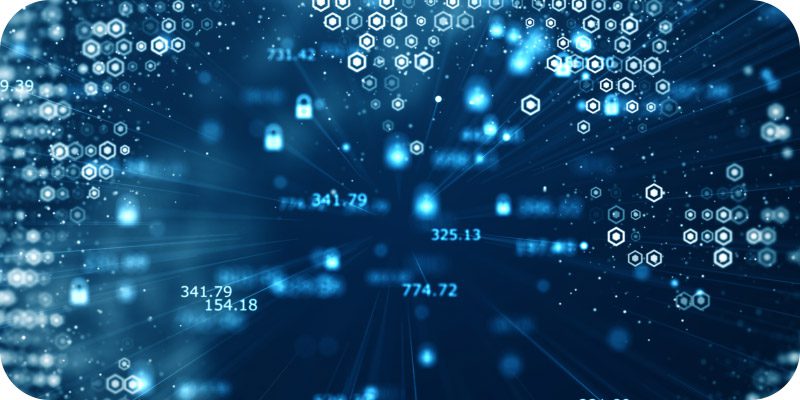
Solutions Review’s Executive Editor Tim King highlights the overarching AI impact on data engineering jobs, to help keep you on-trend during this AI moment.
One of the least surprising things someone can say in 2025 is that artificial intelligence (AI) has impacted data engineering jobs. What is less clear is the specific impact AI has had on those jobs and whether data engineers should be worried. As AI becomes embedded in every stage of the data pipeline—from ingestion to transformation to orchestration—the role and structure of a company’s data engineering team are being fundamentally reimagined.
To keep up with these rapid changes, the Solutions Review editors have outlined some of the primary ways AI has changed data engineering, what engineers can do to stay indispensable, and what the future may look like for the profession and the tools it relies on.
Note: These insights were informed through web research using advanced scraping techniques and generative AI tools. Solutions Review editors use a unique multi-prompt approach to extract targeted knowledge and optimize content for relevance and utility.
AI Impact on Data Engineering Jobs: How Has AI Changed the Data Engineering Workforce?
AI is accelerating a tectonic shift in how data engineering is done. What was once a discipline defined by painstaking, manual construction of ETL pipelines, hand-coded transformations, and endless firefighting of data quality issues is increasingly becoming automated, abstracted, and agent-driven. In many ways, this is a win: repetitive, tedious work is disappearing, and engineers are freed up to focus on architecture, design, and innovation. But there are real risks—especially for those who built careers on legacy tools and traditional best practices. Here’s where AI’s impact is hitting hardest:
Pipeline Automation and Orchestration
Classic data engineering revolved around hand-building and maintaining pipelines. Now, AI-powered orchestration platforms can generate, optimize, and self-heal entire pipelines on the fly, adjusting for schema changes, load spikes, or new data sources with little to no human intervention. For example, tools like Datafold, Ascend.io, and Databricks’ AI Functions can auto-detect dependencies, anticipate failures, and recommend (or even execute) remediations before issues hit production. The upside? Fewer late-night emergencies, faster time-to-value, and increased reliability. The downside? The value of manual pipeline wrangling—once the core of a data engineer’s résumé—is dropping fast. Entry- and mid-level jobs focused on classic ETL are at risk of being fully automated.
Data Integration and Transformation
AI-driven integration tools now connect, map, and transform data across disparate systems with minimal human oversight. Generative AI can write SQL, build transformation logic, and auto-document complex flows—tasks that once required both domain and technical expertise. This lets organizations ingest more sources, more quickly, and handle data drift or changes in real time. But there’s a catch: the margin for error grows as complexity rises, and the risk of “garbage in, garbage out” never fully disappears. While AI speeds up and democratizes integration, human oversight becomes less about doing and more about validating, guiding, and correcting.
Monitoring, Observability, and Data Quality
Data quality issues have always been a thorn in the side of data engineers. AI-powered observability tools (like Monte Carlo, Bigeye, and Soda) can now continuously monitor pipeline health, detect anomalies, and even recommend (or auto-apply) fixes. These systems can predict where problems will occur and flag schema mismatches or failed ingestions without constant human vigilance. The good: this greatly reduces the burden of “break/fix” cycles and shifts engineers toward proactive, preventative work. The bad: some of the traditional “craft” of debugging, troubleshooting, and root cause analysis is being codified and commoditized, shrinking the skill gap between seasoned experts and AI-assisted juniors.
Infrastructure Management and Optimization
With the rise of AI, cloud data infrastructure is trending toward “set-and-forget.” Machine learning algorithms can automatically tune query performance, right-size clusters, and optimize storage with little human tuning required. Cloud providers are already pitching “zero-ops” data platforms, promising massive cost savings and efficiency. For engineers, this means fewer hours spent on low-level tuning and firefighting. But it also means a shrinking need for classic infrastructure and DevOps skills—the sort of “invisible” work that once made a good data engineer indispensable.
A 2024 Gartner survey reported that 62% of organizations using AI-driven orchestration saw a 40% or greater reduction in pipeline maintenance time, while 58% expect to reduce their traditional engineering headcount by 2027. However, 64% reported struggling to hire or retain engineers with the advanced AI, automation, and governance skills required for the next phase of their data platform evolution.
The Emergence of AI-Centric Data Engineering Roles
As with data analytics, the AI wave isn’t just destroying old jobs—it’s creating new ones, fast. Data engineers are being asked to master new platforms and workflows, often acting as the bridge between raw data, AI models, and business outcomes. We’re seeing the emergence of roles like “data automation architect,” “AI pipeline engineer,” and “AI governance lead.” Engineers who can build, prompt, or fine-tune AI-powered orchestration agents will be in high demand—at least for the next several years.
But be critical here: there’s a real possibility that even these AI-fluent roles are transitional. As platforms get smarter and more autonomous, the frontier will keep moving. The only durable advantage will be the ability to deeply understand both data and the business context, to design architectures that are resilient and adaptable, and to guide the ethical and safe use of increasingly autonomous systems. Betting on prompt engineering or “AI agent babysitting” as a career-long moat is, at best, a short-term play.
Upskilling for the Future
If data engineering is your craft, treat upskilling as mandatory, not optional. The old advice—learn one orchestration tool, master SQL, automate what you can—isn’t enough anymore. Instead, focus on:
-
AI and automation literacy: Understand not just how to use AI tools, but how to build, fine-tune, and debug them. Learn how to “think in systems” and design resilient data architectures in a world where much of the day-to-day work is abstracted away.
-
Cloud platform expertise: Stay current with cloud-native, serverless, and zero-ops data stack evolution. Infrastructure-as-code, while still relevant, is morphing fast.
-
Data governance, compliance, and ethics: As AI takes over more “decision-making,” engineers with a handle on lineage, observability, and responsible data management will be invaluable.
-
Communication and business impact: More of your work will be about translating technical possibility into business value, working across teams, and ensuring the systems you build are both powerful and trustworthy.
For organizations, don’t treat AI as a “bolt-on” for existing workflows. The best engineering teams are being reimagined as “platform teams”—builders of the data/AI fabric that enables everyone in the business to move faster, with more confidence.
AI Will Augment Data Engineering Jobs, Not Replace Them—But the Bar Is Rising
If there’s a single thread connecting every conversation about AI and data engineering, it’s this: AI is turning engineers into architects, advisors, and innovators. The “hands-on keyboard” work is vanishing fast. The real value will be in designing the systems, thinking several steps ahead, and ensuring resilience, security, and ethical guardrails. If you’re still writing hand-coded ETL in 2025, you’re swimming upstream.
The impact of AI on data engineering jobs is only accelerating, and the next three to five years will be more disruptive than anything we’ve seen so far. Yes, there’s real risk: the shrinking of classic engineering roles, the loss of “craft” in favor of automation, and the relentless rise of abstraction. But for those who embrace the change—who learn to orchestrate not just data, but the systems that run on it—the future is brighter than ever.
Bottom line: AI will automate the rote, but it will never automate the visionary. To future-proof your data engineering career, focus on the big picture, develop your AI fluency, and become the architect of tomorrow’s data-driven enterprise. The world will always need builders—but the tools and blueprints are changing fast.