Human-Scale AI Value Needs Real-Time Data: 2 Keys to Know
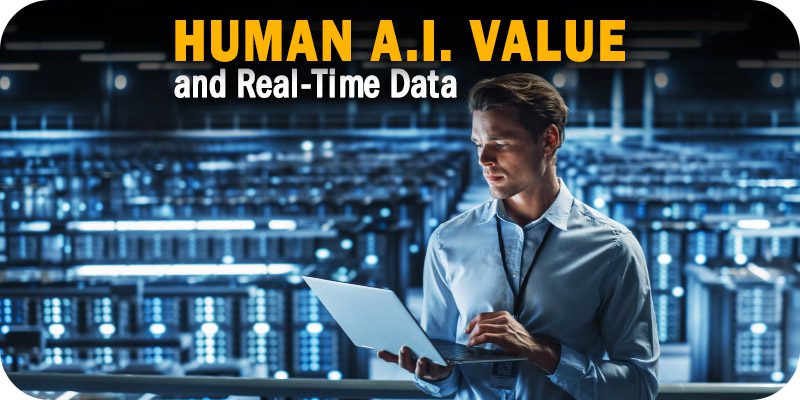
Solutions Review’s Expert Insights Series is a collection of contributed articles written by industry experts in enterprise software categories. In this feature, Arcion CEO Gary Hagmueller offers a compare/contrast on human-scale AI and the value of real-time data, along with two keys to consider.
The AI revolution is upon us. For those of us that have worked in the AI and machine learning space for years, the future is now. The possibility that systems developed today create unprecedented value for posterity is more real than ever before. And the good news is that AI’s potential to generate tangible benefits for adopting enterprises is still in its infancy.
Large language models (LLMs) such as ChatGPT are an amazing advancement that has everyone talking. And as fears of “the rise of the machine” subside with growing familiarity, there is no question that we’re staring at a new era of data-driven AI applications. Concern will likely be replaced with creativity as the diversity of AI techniques becomes more understood and a growing community of practitioners begins to explore the nearly infinite possibilities that AI offers.
As a practical matter, LLMs are successful because they draw on a massive amount of input data. The various generative AI models on the market today have essentially “read” substantial volumes of data from the internet. This gives them the ability to understand complex interaction structures that humans innately use and the content to return (mostly) factual responses. However, the reliance on massive amounts of training data makes these generative applications slow to identify and react to rapidly evolving behavior and choices. While LLMs operate on internet-scale data that changes slowly and trends built over time, enterprises need systems that are extremely sensitive to changes that occur in real time.
It’s likely a foregone conclusion that enterprise applications built on LLMs will become very common in the next few years. However, as LLMs proliferate, it will quickly be obvious to savvy chief data officers that they will always be somewhat general applications. To truly create competitive advantages, LLM-based deployments will need to be augmented with AI applications that leverage the firm’s proprietary data.
Enterprise data is by definition very specific to the company’s ability to generate value. And the greatest generators of enterprise data are the transactional databases that power all functional operations. Equally as pivotal, the most valuable enterprise data is that which was just generated, quite literally.
AI Value and Real-Time Data
Enterprise AI vs. LLM-Based Applications
Enterprise AI will generally take a different form than LLM-based applications. Enterprise AI will typically employ techniques that are more suited to identifying patterns which are subtle, irregular and fleeting — that if identified and paired with an action, generate significant benefit. These methods can be combined with interactive techniques to produce a very rich and highly meaningful application. The nature of this data requires that it be analyzed in as close to real time as possible. Every minute of delay causes data related to a transitory event to lose its value.
The combination of generative and analytical AI techniques offers quantifiable benefits with respect to awesome customer service, exceptional experiences, and substantial operational gains. However, system design is critical to success. To be effective, the elements that must be combined are the ability to interact (which LLMs can solve), knowledgeability (using AI apps built with unsupervised or semi-supervised models address this), and situational awareness (the delivery of real-time data that is rich enough to spot changing phenomena). Situational awareness is a difficult nut to crack as it requires sourcing data from tightly controlled systems that are not set up to deliver a rich analytical experience. However, without situational awareness, AI applications will feel simple, incomplete, or the machine equivalent of an uninformed and unhelpful call center representative.
Accessing Proprietary Data With CDC
The good news is that situational awareness for AI applications is actually within reach. Years ago, database vendors realized that data from operational systems would need to be accessed by other systems. As requirements around high availability, backups, and analytical needs evolved, so too did a form of data replication technology — change data capture (CDC). Of all pipeline-building solutions on the market for transactional data, CDC is the most effective. CDC reads the logs of a transactional database, and as soon as it identifies that a new entry has been committed, it normalizes the row for the format of the downstream (target) system and sends it down the wire in real time. Leading CDC vendors built techniques that guarantee a row is sent once and only once and that entries arrive in the order in which they were committed to the source system. There’s no performance impact on the source system, and security is assured by preventing the downstream consumer from directly accessing sensitive systems. Best of all, by normalizing data on the fly and delivering it in real time, CDC is considerably less resource-intensive and more cost-effective than inferior batch, time stamp, or query-based approaches.
While the first generation of CDC vendors solved the problem of moving data between repositories, the size and complexity of the modern data required by AI applications present a completely new set of requirements that demand a new approach of data replication and migration. Modern CDC vendors have emerged that solve the problem of replicating massive amounts of data in real time. The latest generation of CDC vendors have designed architectures that adopt cloud-based technologies — something that all enterprises are rapidly adopting to manage and conduct business. No truly modern solution would be based on anything other than a distributed microservices architecture that automatically scales up and out with load. Ease of use and intuitive functionality are two other key factors in choosing such a modern data replication solution to ensure you get faster time-to-value.