Small Language Models: The Future of Specialized AI Applications
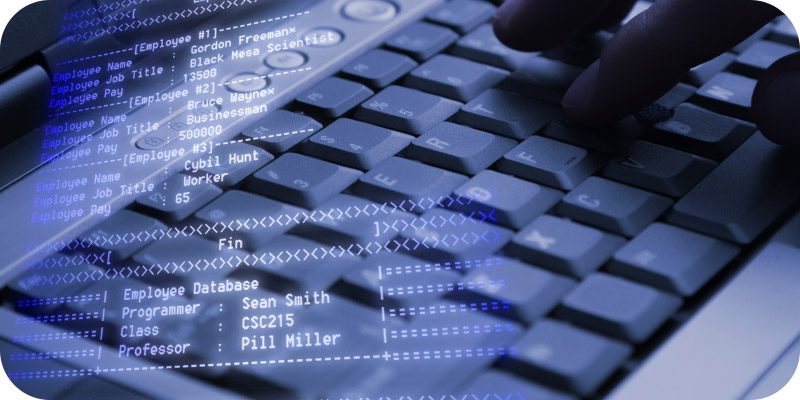
Informatica’s Gaurav Pathak offers insights on small language models and the future of specialized AI applications. This article originally appeared on Solutions Review’s Insight Jam, an enterprise IT community enabling the human conversation on AI.
In the fast-evolving landscape of Gen AI, a new trend is emerging that challenges the notion that bigger is always better. Small language models (SLMs) are gaining traction as a powerful alternative/complement to their larger counterparts, offering a more focused and efficient approach to AI applications. This shift was prominently highlighted at WWDC 2024, where Apple unveiled its latest innovation: Apple Intelligence. This development showcases the potential of SLMs and on-device/edge intelligence.
The Rise of Small Language Models
As the AI industry has matured, it has become increasingly clear that one size does not fit all when it comes to language models. While large language models (LLMs) like OpenAI GPT-4/Anthropic Claude have captured headlines with their broad capabilities, they may often prove to be overkill for specific, targeted applications. This realization has paved the way for the ascendance of SLMs, which offer a more tailored approach to AI development.
Apple Intelligence, introduced at WWDC 2024, exemplifies this trend. By focusing on creating smaller models trained on customized datasets, Apple has designed an AI system that seamlessly integrates into its operating systems. This approach prioritizes practicality and efficiency, ensuring that the AI capabilities align perfectly with the needs of Apple’s user base.
The benefits of SLMs extend beyond just improved integration. These models offer enhanced accuracy within their specialized domains, as they are trained on more focused datasets. This specialization not only improves performance but also addresses critical concerns such as data privacy. By processing data locally on devices, SLMs can offer robust AI capabilities while minimizing the need to transmit sensitive information to external servers.
Moreover, the computational requirements and costs associated with SLMs are significantly lower than those of their larger counterparts. This efficiency makes them ideal for deployment on a wide range of devices, from smartphones to IoT devices, expanding the reach of AI technology.
Parallels with CLAIRE GPT
The approach bears striking similarities to Informatica’s CLAIRE GPT, highlighting a broader industry trend towards more specialized and integrated AI solutions. Both systems aim to seamlessly incorporate AI capabilities into existing workflows and tools, making advanced technology accessible to users without requiring extensive technical expertise.
One key similarity is the focus on recognizing user intent and providing context-specific responses. By leveraging smaller, task-oriented models, both Apple Intelligence and CLAIRE GPT can quickly understand what the user is trying to achieve and offer relevant assistance. This approach stands in contrast to more general-purpose AI systems that may require additional prompting or clarification to hone in on the user’s specific needs.
Integration is another critical area where these systems align. Apple Intelligence is designed to blend seamlessly into the operating system, becoming an almost invisible yet powerful assistant across various applications. Similarly, CLAIRE GPT is engineered to be available across a range of data management applications, providing consistent AI support throughout the Informatica Intelligent Data Management Cloud.
The emphasis on specialized, task-oriented AI in both systems reflects a growing industry trend toward making AI more practical and immediately useful in everyday scenarios. Rather than attempting to create a single AI system that can do everything, the focus is on developing multiple specialized models that excel in specific domains or tasks.
Transparency, Interpretability & Explainability
As AI systems become more integrated into our daily lives and critical business processes, transparency, interpretability, and explainability will become important. Both Apple and Informatica recognize this crucial aspect in their approaches to AI development.
Transparent AI practices are becoming increasingly important as consumers and regulators demand greater insight into how AI systems make decisions. This transparency is not just about complying with regulations; it’s about building trust with users and ensuring that AI systems can be reliably deployed in sensitive environments.
Interpretability refers to the ability to understand how an AI system arrives at its conclusions. This is particularly important in domains where decisions can have significant consequences, such as healthcare or finance. By using smaller, more focused models, systems like Apple Intelligence and CLAIRE GPT offer greater interpretability than larger, more opaque models.
Explainability goes hand-in-hand with interpretability, focusing on the ability to provide clear, understandable explanations for AI decisions. This is crucial for building user trust and enabling effective human-AI collaboration. Both Apple and Informatica appear to be prioritizing this aspect in their AI development strategies.
The Importance of Data Foundations
While the shift towards SLMs and specialized AI applications is promising, it’s important to recognize that there are no shortcuts in AI development. The quality and governance of the underlying data remain paramount, regardless of the size or specialization of the model.
To truly deliver on the promise of AI, applications must be built on holistic, high-quality, and well-governed data foundations. This includes implementing robust data access management and privacy controls from the ground up. Without these foundational elements, even the most advanced AI models will struggle to provide reliable and valuable insights.
Furthermore, as AI systems become more deeply integrated into critical processes, the need for adequate data management becomes even more crucial. This includes ensuring data accuracy, maintaining data lineage, and implementing strong data security measures. These practices not only improve the performance of AI models but also help in meeting regulatory requirements and building user trust.
Conclusion
The emergence of small language models represents a significant shift in the AI landscape. By focusing on specialized, task-oriented AI, these systems offer improved accuracy, enhanced privacy, and more seamless integration into existing workflows.
However, the success of these AI applications ultimately depends on the quality and governance of their underlying data foundations. As we move forward, the focus should be on developing AI systems that are not only powerful and efficient but also transparent, interpretable, and explainable.
The future of AI lies not in creating ever-larger models but in developing specialized, well-governed systems that can be seamlessly integrated into our daily lives and business processes. As Apple, Informatica, and other industry leaders continue to innovate in this space, we can expect to see AI becoming an increasingly invisible yet indispensable part of our technological ecosystem.