Data Lakehouse Architecture: Key Advantages for Modern Firms
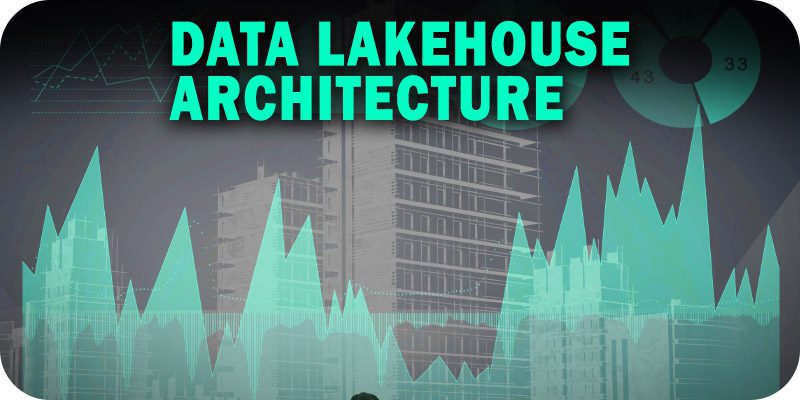
This is part of Solutions Review’s Premium Content Series, a collection of contributed columns written by industry experts in maturing software categories. In this submission, Dremio Vice President of Product Mark Lyons offers an overview of data lakehouse architecture, and how the approach is a strategic advantage over traditional architectures.
For years, executives have spent time and resources evaluating the best ways for organizations to become data-driven. The end goal has always been to take corporate data and enable deeper insights into business functions and customer behavior. This became widespread with the advent of the data warehouse, and we are now seeing organizations evolve with the next iteration of data architecture—the data lakehouse.
Understanding the Limitations of Traditional Data Architecture
Traditionally, the data warehouse has been used as a centralized repository for corporate data. Its use has been the standard protocol for data management. There are two groups of power users: data providers and data consumers. The data providers are data engineers, application developers, and data architects. Business analysts, data scientists, and executives make up the data consumers.
Data warehouses have been great for integrating data across organizations, and when combined with other semantic and business intelligence (BI) technologies, they provide tangible value to data consumers. But delivering data products efficiently has been a challenging problem to tackle with a data warehouse due to the complexity of getting quality data into the hands of the consumers.
When data consumers request more data, they get put into a queue. There is a waiting period before the data gets updated. Depending on the request, this can take days, weeks, or even months.
Under the hood, data engineers are building and maintaining complex ETL (extract, transform, and load) processes for new data. They are overwhelmed with competing data requests and ensuring current pipelines are not failing. Here’s how the conventional ETL process works:
- Source application data is loaded into a data lake for cheap and scalable storage.
- Raw data is cleaned and normalized, then copied into a proprietary format in the data warehouse.
- Corporate data in the warehouse is extracted into layers of data marts and transposed into a semantic layer for self-service reporting across departments.
- Any data modifications, such as slowly changing dimensions, will need to be reworked upstream in the ETL pipeline before pushing to production.
With growing workloads, the data warehouse is limited to processing structured data. Given the complex ETL processes, the rigidity of a warehouse makes it an inflexible analytics solution with changes in business requirements. Data engineers end up extracting data out of the warehouse into BI cubes for self-service analytics. This method is expensive and time-consuming.
Data movement through a monolithic data infrastructure is like activity in a kitchen with no cookware. Data engineers struggle with data prepping if the kitchen does not have the proper cookware to handle the growing volume, velocity, and variety of data. The data warehouse locks data into one engine, preventing you from using the right tools for the right workloads. Without the right tools, data engineers will spend most of their time making sure pipelines aren’t breaking. By the time data is served, business requirements may have changed.
What organizations need is a simplified data infrastructure that reduces the complexity and costs of data copying. Data teams want to focus on driving business outcomes with accurate information for decision-making. A data lakehouse enables this, and most importantly, creates an easy path for self-service analytics with the necessary data management and governance.
Bringing Users to the Data With a Semantic Layer
Recently, the biggest shift in data management infrastructure has been the adoption of the data lakehouse, a layer with the benefits of both data lakes and data warehouses. The data lakehouse is based on an open-table format architecture like Apache Iceberg, so teams can use any engine of choice to access data on the lakehouse. Data is stored in the data lake—which includes a semantic layer with key business metrics—all realized without the unnecessary risks of data movement.
For organizations that want to democratize data with a data lakehouse, the semantic layer is an essential component. Some of the benefits include:
- Customized views of data for different functional organizations,
- Consistent business metrics, and
- Self-service analytics with BI tools of choice, and
- Enforced data governance and data quality across teams.
With a semantic layer built directly on the data lakehouse, both data providers and data consumers get the hidden benefit of increased productivity. Data engineers don’t spend as much time modifying brittle ETL pipelines. They get more bandwidth to work on high-value projects and deliver more data products. Data reproducibility becomes a reality, empowering data consumers with self-service analytics. Democratization of data directly on the data lakehouse promotes collaboration across teams and offers a governed layer for analysis.
If a monolithic data warehouse is like a kitchen without proper cookware, then a data lakehouse semantic layer is like a professional kitchen in a five-star restaurant. ETL pipelines are simplified because the chefs are operating at their highest level of efficiency. The complexities that come with growing volumes of data, such as data management and governing data access requests, are reduced. End-users can enjoy the data without worrying if the data has been prepared correctly.
Data is everything to a business and market conditions have forced companies to change their data management strategies. Many organizations are now realizing the high cost of copying data in and out of a data warehouse. Being efficient with how they operationalize their data is a business necessity. With a lakehouse, everything lives in your data lake. Instead of bringing your data to the engine, you bring the engine to the data.