Key Enterprise Knowledge Graph Examples for Data Quality Success
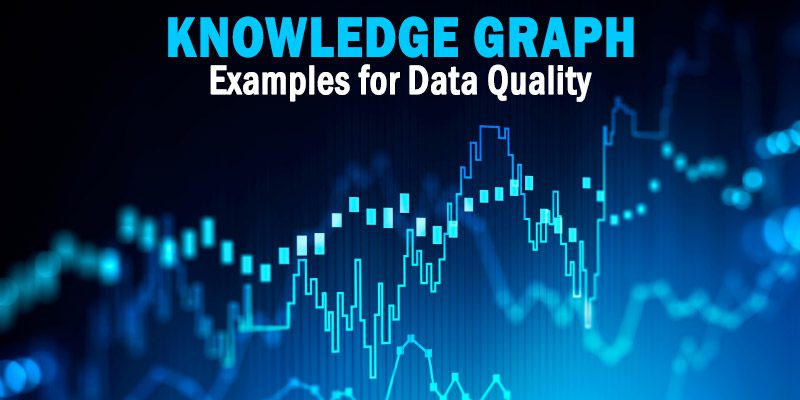
This is part of Solutions Review’s Premium Content Series, a collection of contributed columns written by industry experts in maturing software categories. In this submission, Stardog Solutions Consultant Tim Sedlak offers key enterprise knowledge graph examples for data quality success.
The amount of unstructured and structured data continues to grow exponentially and the deluge shows no signs of letting up. Despite efforts to find more effective ways to store and manage these critical assets, businesses still continue to struggle with significant, enterprise-wide data proliferation and the data mess that often results. It goes without saying that the ability to unlock the power of data is often the key to a business’s success. But finding the necessary data to achieve this advantage is becoming increasingly difficult in today’s complex data stack.
The digital transformation movement has only exacerbated the problem due to the increased demand for highly curated datasets to support advanced analytics, machine learning, and other applications. Data quality, while always important, has become even more critical because enterprises lose out when they are forced to rely on incomplete or inaccurate information sourced from disparate, disorganized, and rapidly changing data sources.
Options for Achieving Data Quality
Organizations continue to search for a magic bullet to cure their data quality troubles. But the reality is that dirty data can occur in a number of ways. Invalid fields, missing and/or additional values, consistency issues such as duplicate data, etc. are the usual suspects. As a result, organizations tend to look at solutions such as Master Data Management (MDM) to establish a single source of truth for data domains across an organization or through traditional data quality tools to identify, understand, and correct issues in the data. The problem is that each of these approaches require a significant investment to build and are often unable to keep up with the organization’s evolving data management landscape.
Thanks in part to the increasingly hybrid, varied, and changing data environments, it’s increasingly common for organizations to augment MDM strategies with tools that can easily represent multiple versions of the truth. Solutions such as an Enterprise Knowledge Graph (EKG) are one of them, as it allows the business to rid themselves of dirty data, amplify their MDM efforts, and future-proof the effort across both existing and future environments.
This is because EKGs are purposefully designed to simultaneously support different use cases, orgs, lines of business, and apps in sharing and reusing connected data. Supporting the dynamic delivery of semantically enriched data, these EKGs use a unique combination of virtualization, inference, and data quality validation, making them an ideal choice for dealing with dynamic, disparate, and even messy, data.
Enterprise knowledge graphs are also considered a key component to transforming an enterprise’s data infrastructure into a modern data fabric, which Forrester defines as a hot, emerging market that delivers a unified, intelligent, and integrated end-to-end platform to support new and emerging use cases, delivering them quickly by leveraging innovation in dynamic integration, distributed and multi-cloud architectures, graph engines, and distributed in-memory and persistent memory platforms.
This is because EKGs can simultaneously support different use cases, orgs, lines of business, and apps in sharing and reusing connected data. Also, when used as part of a data fabric, it can create a single, reusable data foundation that can power multiple applications, even if data has to be defined differently across use cases.
Using Enterprise Knowledge Graphs to Establish a Responsive Data Approach
Multiple schemas, or data models, are required to manage an enterprise. Rather than being stuck with reactive data management strategies and degrading data quality, enterprises now realize that they require a more responsive data approach in order to keep pace with the needs of the business and are applying EKGs to leverage functionality such as:
Virtualization and Data Integrity
Organizations can retrieve and manipulate data without having to know details such as how it is formatted at source or where it is physically located. Data virtualization (DV) provides a cost-effective alternative to traditional and expensive data integration techniques that require data to be replicated, moved, and stored multiple times. It removes the need to copy data for every new project or use case which eliminates data drift and errors.
DV creates a single source of trust for end-users by guaranteeing that the data consumed is the most current and accurate. Errors discovered in the data can be directed to the source system owners and subject matter experts for correction and, once addressed, be immediately reflected in the results seen by end-users because the data was never persisted in the knowledge graph itself.
Inferencing and Data Correctness
By associating related information that may reside across disparate sources and applying business rules based on a semantic data model, organizations can discover new connections along with additional insights. By combining relationships and rules at query time, organizations achieve a richer, more complete, and more accurate view of the data. Not to mention the ensuing insights are up-to-date.
Constraint Validation and Data Reliability
This feature enforces data integrity and helps improve the correctness and consistency of the knowledge graph itself. It validates data according to constraints described by users and what makes sense for their domain, application, and data.
These constraints can also identify inconsistencies across data sources, flag data conflicts, and even prevent the knowledge graph from accessing corrupt data in the first place. These constraints help measure the quality of the data, perform verification after integration, and assist in planning future improvement measures. They can also offer explanations for constraint violations and provide insights into what the invalid data is and why it is unacceptable.
Final Thoughts
At the end of the day, to be truly useful to an organization, the data itself must be valid and consistent. Enterprise knowledge graphs can help enforce data integrity, improve data correctness, and ensure data consistency. With these safeguards in place, organizations and end-users can clean up their data and rest easy knowing they are receiving complete and accurate results that they can use to solve their business needs.