What is Data Observability and How Does it Improve Data Quality?
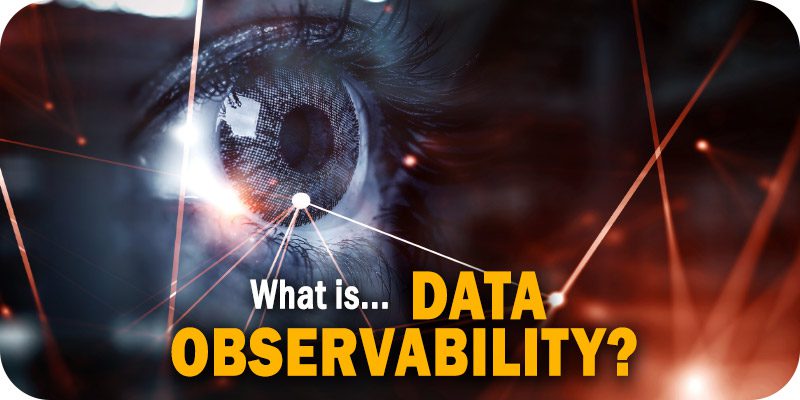
This is part of Solutions Review’s Premium Content Series, a collection of contributed columns written by industry experts in maturing software categories. In this submission, Kensu Founder and CPO Andy Petrella answers the question “What is Data Observability?” and offers some key ways it helps to improve data quality.
Data quality issues significantly impact thousands of organizations every day. Among others, low data quality can break automated reports with figures that do not add up, or they can lead to ML models producing poor recommendations. These result in poor user experiences or wrong business decisions including investment in the wrong sectors, reducing production or capacity rather than increasing it, and a multitude of other issues that come with high price tags.
Gartner research in 2018 found organizations estimated poor data quality to be responsible for $15 million per year in losses. A recent D&B survey found that 1 in 5 businesses lose revenue and customers due to incomplete data. As businesses become increasingly data-driven today, and they are collecting and processing more and more data, this will only lead to more frequent data quality issues and costly missteps.
Over time, these issues also lead to a drop in confidence in the data, causing the decision-makers to be cautious when acting on it and show less enthusiasm when deciding the budget allocated to the data department.
When these data issues and their consequences rise to the surface, sometimes, after having impacted the business for weeks or months, data teams must locate and troubleshoot them. This exercise can take hours, days, and sometimes even weeks as multiple stakeholders might be involved: those who collect the data, those who process it, and finally, those who consume it.
Patiently the data team will have to dig in and explore the pipelines and their applications, wasting their time while enduring some backfire from the business users. Sometimes, they will be able to find the root cause and adequately fix the problem; sometimes, they will run out of time and implement some quick fixes, which will probably cause more problems in the future.
Know Your Data at its Source
A solution to these problems is to observe the data directly into the pipelines, where and when it is being processed. This approach, called data observability, is achieved by automatically:
- Recording where the data comes from
- Monitoring the quality of data (e.g., distribution, volume, average)
- Logging how the applications process it
- Documenting where the data goes
This information helps data teams understand their data pipeline health and address, in no particular order, the leading causes of data incidents:
- Inconsistency
- Inaccuracy
- Incompleteness
- Obsolescence
Most organizations have to deal with multiple, simultaneous data quality issues. They might have too many different data sources with a combination of the four of the top causes of these issues. All this takes time and effort to resolve, both typically in short supply. Including observability in the process will greatly reduce the occurrences of data quality issues.
Data observability is the ability of a data system to provide enough information about its behavior so that an external observer can interpret its status. And it is growing in favor. In 2022. Gartner included it in the Gartner Hype Cycle for Emerging Technologies which features 25 “must-know innovations” to drive competitive differentiation and efficiency.
Data observability solutions allow rules to be set that will trigger alerts when something unexpected happens along the data pipeline. This allows data teams to address issues in a timely manner before they propagate through to dashboards or models, and then on to uninformed decision-making.
All this ‘observability’ information and infrastructure empowers those responsible for data pipelines, typically data engineers, to deliver data to quality and on time. If there is a data issue, the data engineers will be the first to know about it, not the consumers of dashboards or models.
The Power of Data Observability
Including observability into the data infrastructure can help minimize the number of data issues and help data teams bring about a swift resolution to questions that do arise.
Along with some form of observability implementation, best practices that the most successful data-driven teams have also implemented include:
- A process and data-driven culture
- Automation of as many tasks as possible to reduce errors
- Deployments are automated and executed only when automated tests are passed
The benefits of data observability go beyond providing visibility. It gives data teams the ability to scale their data ecosystems, improve productivity, and collaborate better with other data teams. For a more detailed understanding of the approach, please check out The Fundamentals of Data Observability published by O’Reilly.