Key Takeaways from Gartner’s 2019 Market Guide for Data Preparation
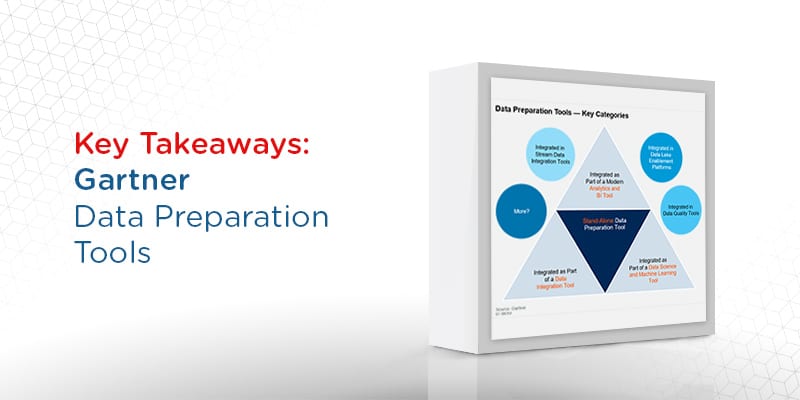
Analyst house Gartner, Inc. recently released its new Market Guide for Data Preparation Tools. The researcher’s Market Guide series is meant to cover new and emerging markets where software products and organizational requirements are in limbo. Gartner’s Market Guides can be a great resource for understanding how a fledgling space may line up with current and future technology needs.
According to Gartner, “With the focus on early, more chaotic markets, a Market Guide does not rate or position vendors within the market, but rather more commonly outlines attributes of representative vendors that are providing offerings in the market to give further insight into the market itself.”
Though Gartner’s Market Guide is not provider-centric and instead, aims to provide a more overarching view of the software space, the research house does mention the vendors to watch as things take shape. Many of the solution providers included are major players in vertical markets, such as BI and data analytics and data management.
Gartner highlights the following providers in data preparation: Altair (formerly Datawatch), Alteryx, Cambridge Semantics, Datameer, Elegant MicroWeb, erwin, Infogix, Lore IO, Modak Analytics, Paxata, Rapid Insight, SAP, SAS, Talend, Tamr, TMMData, Trifacta, Unifi, and Yellowfin. At Solutions Review, we read the report, available here, and pulled out the key takeaways.
Data preparation is widely regarded as one of the most time-consuming practices in the analytic process. As a result, Gartner proceeds with the stance that preparing data for analysis is changing from an end-user dominated self-service function to an “enterprise imperative.”
This marketplace is unique because it encompasses providers, technologies, and software products from other vertical markets, with many of the top vendors including data preparation capabilities inside their existing BI and analytics, data science, and data integration solutions. In this way, data preparation tools lack definition. However, an increasing number of enterprise technology providers are expected to follow the footsteps of forward-thinking vendors in releasing standalone products.
Gartner recommends data and analytics leaders create a data preparation deployment strategy that will “enhance user understanding of data, reduce data preparation efforts and increase agility.” Organizations should evaluate software in this space based on capabilities like connectivity, machine learning-based automation and data cataloging to improving searchability among their data assets. When a data preparation use case is general purpose in nature, standalone data preparation tools are likely best, with embedded functionality relevant in settings are more complex.
Gartner’s research leads the analyst to believe that data preparation will become a critical part of data integration, analytics, and BI in just three years. It is their opinion that these capabilities will be commonplace in data integration projects for analytics and data science as well. However, investing in standalone products may be risky due to the fact that data preparation features are quickly being added to solutions in other markets, namely by data management providers.
The data preparation space is divided into four distinct segments, including features integrated as part of modern BI, integrated as part of data science and machine learning, integrated as part of data integration, and standalone offerings. Your mileage will vary, and the tool that is right for your organization will largely be decided by your goals and current technology environment. It will also be dependent on whether your use case is basic or complex.
Gartner believes that there are 7 key (or critical) capabilities that make up a modern data preparation offering. We believe this list will continue to expand as demand for clean, secure and actionable data proliferates further in the enterprise. For these insights and more, we highly encourage you to read the complete report linked below.