The Rise of Specialized LLMs: Tailoring Generative AI for Enterprise Excellence
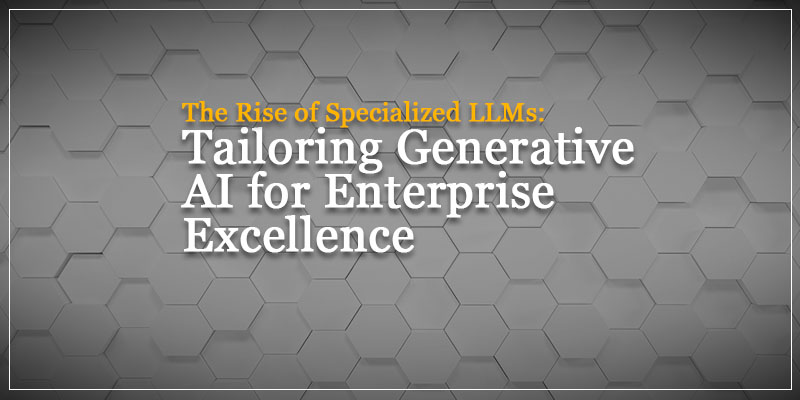
As part of Solutions Review’s Contributed Content Series—a collection of contributed articles written by our enterprise tech thought leader community—Raghu Ravinutala, the CEO of Yellow.ai, explains how specialized LLMs can help enterprises utilize generative AI in the best way.
It must be said that generic large language models (LLMs) such as GPT-4 may not be the most appropriate and effective for enterprises. Another way to put it? Without training LLMs on a local “domain-specific” text corpus, enterprise applications are highly likely to yield suboptimal results. That’s because Generalized AI models that are built on massive data sets with the ability to respond in a perceived human-like conversation often lack the nuance and specificity needed for enterprise-specific use cases.
Expanding the LLM’s parameters is also not a practical approach to enhancing its results. The current one-size-fits-all generative AI model has led to more hallucinations, generic outputs, poor integrations, and vulnerabilities. Businesses that are trying to implement generative AI require technology that is tailored to their specific needs, industry terminology, and unique personalities.
How to NOT Implement Generative AI
Companies of all sizes want to harness and integrate generative AI into their business practices to streamline the worker and customer experience. 79 percent of business leaders predict their employees will significantly utilize generative AI in their weekly work. McKinsey has pinpointed 63 potential generative AI applications across 16 different business functions, estimating that these could generate economic benefits ranging from $2.6 trillion to $4.4 trillion annually when deployed across various industries. That’s precisely why organizations are racing to implement this technology, striving to maintain their competitive edge. Those who overlook its integration are risking being left behind by their competitors.
However, rushing the implementation of generalized AI models that don’t cater to specific enterprise use cases or functions can often lead to various issues, including hallucinations, high latency, and unreliability. Any company haphazardly embracing giant LLMs has failed to consider why it needs AI and how it plans on using it. Additionally, it’s essential to consider the potential consequences of this kind of rapid expansion, as it can result in the depletion of resources and scalability challenges.
So, how do companies take advantage of this ‘must-have’ technology? The Solution: Specialized-LLMs.
Unique Solutions for Unique Business
A recent report states that 70 percent of organizations are exploring and investing in generative AI research to eventually incorporate it into their business practices. Large tech companies like Google, Microsoft, and Meta are developing their own proprietary, customized language models to provide a unique and personalized experience to their customers. When dealing with language models, opting for smaller, specialized variants that undergo modifications such as domain-specific pre-training, model alignment, and supervised fine-tuning for particular purposes can lead to a reduction in hallucinations and enhanced accuracy.
Specialized LLMs are trained on industry-specific knowledge and can understand technical language and concepts. This fundamentally makes utilizing these models more practical and impactful in achieving business outcomes. However, not all companies have the resources to create specialized LLMs like these tech giants. This is where third-party vendors come in.
Companies with a focus on AI are in the process of creating next-generation models tailored for particular use cases. These models trim away unnecessary parameters and offer specialized Large Language Models (LLMs) geared toward functions like customer support, marketing, sales enablement, or specific industries such as retail, e-commerce, and finance. These specialized LLMs are readily accessible for enterprise usage. This approach results in an improved, efficient, and personalized experience, making AI models more effective and accessible.
Where We’re Headed
Looking ahead, the future seems bright and optimistic. In 2023, AI models have already advanced to a level where they can generate content and responses that are almost indistinguishable from those created by humans. For instance, dynamic AI agents powered by these specialized LLMs have made it possible for businesses to automate customer interactions that were previously impossible.
As a result, companies have seen a 40 to 50 percent reduction in service interactions, increased customer satisfaction, and faster resolution times. Brands can now engage in longer and more meaningful conversations with customers thanks to these new breeds of agents. This increased productivity has and will continue to translate into significant cost savings (up to $80 billion by 2026) and growth for businesses of all sizes.
How to Attain This Generative AI-Powered Productivity
When preparing to integrate generative AI, the process begins with a clear definition of objectives and the collection of relevant data. The second crucial step involves developing an in-house solution powered by a specialized LLM or partnering with an automation provider that offers specialized LLM-powered solutions tailored to the enterprise’s specific use cases or focus areas. This choice includes selecting an appropriate AI model and fine-tuning it with domain-specific information, which not only enables cost optimization but also minimizes hallucinations and enhances efficiency.
Once the solution is seamlessly integrated into the workflows, the subsequent steps involve continuous monitoring and maintenance to ensure optimal performance. Additionally, providing user training is essential to maximizing the potential benefits of the technology. Establishing a feedback loop facilitates ongoing improvement. It is of utmost importance to maintain a strong focus on data security and compliance, adhering to relevant industry standards and regulations. As positive outcomes are realized, the enterprise should contemplate the possibility of scaling up AI adoption across various functions and applications, leveraging the lessons learned and experience gained in the initial stages of implementation.
Whatever the plan of action, companies must strive to improve their user experiences and overall operations, or they risk losing talent, consumers, and, eventually, their business. Implementing specialized generative AI solutions is one fundamental way to remain competitive, unique, and relevant in 2023 and beyond. This requires a personalized approach that caters to the individual needs of each organization. By doing so, business leaders can create a one-of-a-kind experience that sets them apart from their competitors and takes their company to the next level.