Change Data Capture: The Top 5 Use Cases
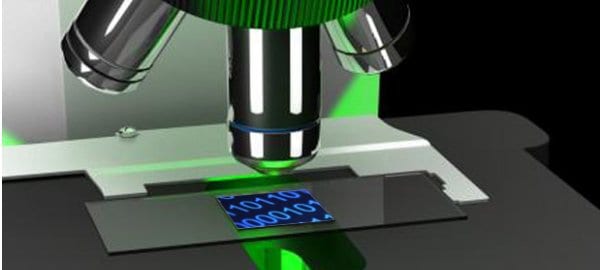
As data volumes increase and data latencies fade away, the traditional bulk processing approach to data integration is “doomed to fail” according to Itamar Ankorian – Director of Marketing at Attunity.
In an article at TDMI.org, he argues for a new data processing approach, “The solution of choice is to change the processing paradigm and only work with data that actually changed, which in many cases is a fraction of the source data (e.g., 5 percent). This paradigm is based on change data capture (CDC), a technology that reduces costs and enables improvement of data timeliness, quality, and consistency.”
In his post, Mr. Ankorian outlines the Top 5 Change Data Capture use case applications that can benefit from this new line of thinking.
- ETL for Data Warehousing: “The most common case for CDC is in loading data warehouses, where processing changes can dramatically reduce load time, required resources (e.g., CPUs, memory), and associated costs (e.g., software licenses). In many cases, daily changes represent a fraction of the total data volume, so CDC has a big impact on efficiency and provides a solution for the continued and accelerating growth in data volumes.”
- Slowly Changing Dimensions: “Any data warehouse team needs to address slowly changing dimensions (SCD), which requires identifying the records and attributes that changed. For large dimension tables, this is a demanding and inefficient process, typically done by joining staging and production tables.”
- Data Replication for BI: “As reporting and BI become more pervasive in supporting daily operations, more users require access to timely information from their production systems. A common solution is to offload production data to a secondary database that is then used by operational reporting applications.”
- Master Data Management: “Key objectives of any master data management (MDM) initiative are to improve and ensure the quality and consistency of master data, whether stored in a single repository or distributed across many. This requires timely responses to master data changes.”
- Data Quality: “Improving data quality in source systems has become a common requirement, typically implemented by periodically scanning the data. By capturing and processing only changes, CDC enables ETL jobs (used to clean data) to run more efficiently and frequently. As a result, errors can be corrected faster, improving decisions and operations.”
A more detailed post is available at TDWI.org.
Widget not in any sidebars