Best Practices for Data Enrichment After ETL
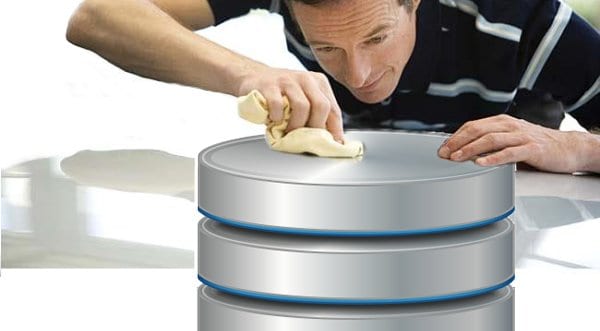
Bob Lambert, a Director at CapTech Consulting, has over 25 years experience in data warehousing, data management, project management, and application development. In a recent post on Smart Data Collective, Mr. Lambert offers some great advice on the concept of Data Enrichment. He begins with a unique premise, “The data integration process is traditionally thought of in three steps: extract, transform, and load (ETL). An additional step, data “enrichment”, has recently emerged, offering significant improvement in business value of integrated data. Applying it effectively requires a foundation of sound data management practices.”
According to Mr. Lambert, “Data integrators traditionally bring data from source to target unchanged. It’s as if ETL developers were movers who prided themselves on putting your furniture in the new place unbroken. Businesses today are asking the movers to repair and improve the furniture before landing it in the new house.”
So he has offered a list of some types of information that can be augmented to a demographics database like:
- “Geographic: such as post code, county name, longitude and latitude, and political district
- “Behavioral: including purchases, credit risk and preferred communication channels
- “Demographic: such as income, marital status, education, age and number of children
- “Psychographic: ranging from hobbies and interests to political affiliation
- “Census: household and community data”
Mr. Lambert also offers three guiding principles for organizations adding enrichment to their data integration streams:
- The business should drive and manage enrichment definition: Data stewards who understand the incoming data and the intended use must be the key drivers of what data is enriched, how it is done, and test of the enrichment outcomes.
- Enriched data must be identifiable and audit-able in the target database: Any integration target database should feature complete lineage metadata: where is this data element from, when was it loaded, and what happened to it along the way. This is even more true for data added by interpolating from, augmenting, matching, or correcting source data. Analysts must know which data came directly from the source, which was generated, and the confidence level of the latter.
- Data replaced by enrichment must be available alongside the enriched data: Enrichment processes must store modified or added data in such a way that analysts have access to the “raw” source data. Analysts should be able to independently test enrichment processes and suggest improvements if needed. If, for whatever reason, enrichment doesn’t meet specific analysis needs, then they should be able to fall back to the original source data.
A link to the post can be followed here.
Widget not in any sidebars