Data Observability vs. Data Monitoring; What’s the Difference?
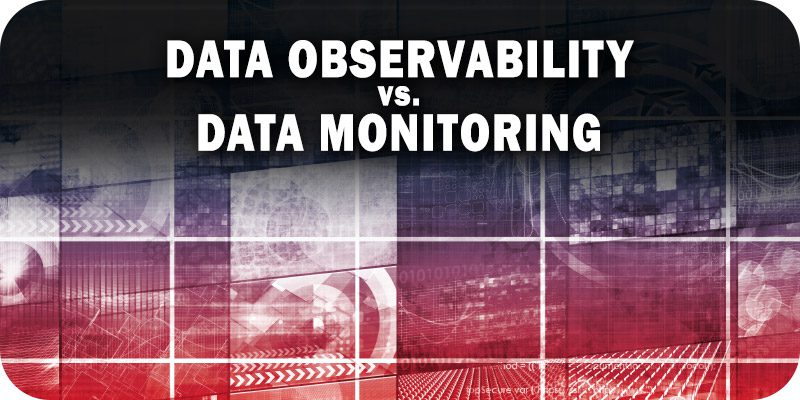
This is part of Solutions Review’s Premium Content Series, a collection of contributed columns written by industry experts in maturing software categories. In this submission, EPAM Systems‘ Senior Manager of Data Analytics Consulting Petr Travkin compares data observability vs. data monitoring so you know the difference.
Data is the currency of the modern world. And Consistent collecting and interpreting quality, trustworthy, and up-to-date customer information can mean the difference between winning and losing in business today. But, with around 2.5 quintillion bytes of data collected by organizations every day, sifting through this unimaginable ocean can be challenging – not to mention overwhelming. Moreover, poor data quality, or data that is partial or incorrect, can result in wasted time and resources.
For companies that recently migrated to the cloud, have complex data stacks, or constantly experience data quality issues, it is time they implemented a data observability strategy.
What is Data Observability? Data observability is a DataOps process that helps data teams ensure high data quality, giving them the transparency to identify issues and the control to resolve them at the point of occurrence rather than further downstream when damage is irreversible. Observability tools and procedures enable data experts to detect, resolve and prevent data anomalies. From a broader IT context, observability is a company’s ability to understand and manage the performance of all of its systems, servers and applications.
Fundamentally, this is a measurement of the state of internal systems based on the available information from external outputs.
The term observability has existed for many years. The modern interpretation comes from software observability, where businesses gather data from program execution, the internal states of modules, and communication between components to determine if everything is performing as expected. Simply put, observability is an evaluation of the state (good or bad) of internal systems based on information obtained from outputs.
Data Observability vs. Data Monitoring
How Does Data Observability Differ from Data Monitoring?
Data observability and monitoring are frequently used interchangeably with each other. While it is not entirely accurate to say that they are the same, there are overlaps between the two, and sometimes they work in conjunction. Likewise, data monitoring and observability serve similar functions as they facilitate the collection of diverse data sets to help businesses recognize problems within their IT landscape.
Nevertheless, there are important differences. Namely, data monitoring can only alert a data specialist to an issue; it can only tell someone if something is broken, but not necessarily how to fix it. Observability, however, can detect and provide details about the source of the problem. During the emergence of early IT systems, businesses did use data monitoring. But because of the ever-expanding and increasingly sophisticated nature of software and infrastructure, companies needed better visibility capabilities, leading to the reliance on data observability.
Another key distinction between data observability and monitoring concerns whether the data pulled from an IT system is or is not predetermined. Data monitoring typically anticipates issues or irregularities using established criteria to measure against perceived problems. On the other hand, data observability collects metrics across the entire IT landscape to proactively discover possible abnormalities.
It also leverages machine learning (ML) techniques to not only identify the problem but learn what went wrong. And because data observability measures all the outputs across multiple applications and systems, it can offer actionable insights into the IT landscape’s health, which data monitoring cannot do.
The Benefits of Data Observability
As already discussed, data processes and structures have grown in sophistication, importance, and size. With more data sources, faster data flows, and higher complexity of transformations, there is more surface area to maintain but less time to implement fixes without creating a significant impact. Likewise, there is always a trickle-down effect whenever there is a breakdown in a data pipeline, as every use case that depends on that data no longer functions properly. Ultimately, a brand’s final product or service suffers, eroding customer trust.
Data observability provides data teams visibility into their ever-expanding and accelerating data landscape, enabling them to effectively measure the health and usage of data within pipelines, including health indicators of the overall ecosystem. By utilizing artificial intelligence and ML-powered solutions, like automated logging and tracing, data observability interprets the health of datasets and pipelines, allowing engineers and analysts to quickly spot changes in the origin, integrity, and availability of the data across the organization.
Data observability also helps teams rapidly resolve issues at their root, improving pipelines, boosting productivity, and creating happier customers and stakeholders. Additionally, a best-in-class data observability platform will integrate quickly and seamlessly with existing data stacks without any rip-and-replace producers or disruption to pipelines.
Data observability is central to establishing and sustaining data governance. A proper data governance program is inseparable from successful data quality management. Without data governance, collaboration becomes difficult as teams will speak a different language with dissimilar definitions. Data governance is also critical to context; a lack of context makes it challenging to organize, evaluate and extract insights from data. Unfortunately, many businesses enforce data governance through inefficient and outdated tooling methods. However, companies can promote better data governance practices by deploying data observability tools.
DataOps and The Future of Data Observability
Data observability remains a relatively new field in the data space – with time, these solutions will evolve to become even more valuable. And, when combined with tested DataOps practices, brands will use data observability to refine and enhance capabilities, improving the trustworthiness of data pipelines, creating more reliable workflows, or stimulating data-driven innovation. Indeed, as long as enterprises base their decisions on data, observability will be indispensable.